Editor’s Introduction: What AI Can and Can’t Do and How Psychoanalysis Can Help
by Alexander Stein, PhD
8-10 min
“I propose to consider the question, ‘Can machines think?’”
So began Alan Turing’s ground-breaking 1950 paper “Computing Machinery and Intelligence.”
By 1956, John McCarthy, a computer scientist at Stanford University, was convening a conference at Dartmouth College attended by many of the leading minds in computer science at the time. These researchers would take up Turing’s provocative question to explore the potential for developing intelligent computer systems based on logic and problem-solving that could simulate human thinking and intelligence. McCarthy is credited with first introducing the term Artificial Intelligence, which he considered “the science and engineering of making intelligent machines” that could perform “the most advanced human thought activities.”
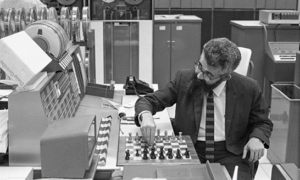
John McCarthy plays chess against a computer | photo: Chuck Painter, 1966
Seventy years later, the answer to Turing’s question remains inconclusive. In certain quarters, Artificial General Intelligence (AGI) is nearly within reach while many claim the goal of machine intelligence has already been achieved as evidenced by the proliferation of mass-market AI services and applications in use today. Others remain skeptical about the possibility of computational systems ever overcoming the multiple technical barriers required to achieve human-like intelligence. And then there are those like futurist Ray Kurzweil who benchmark progress against the establishment of The Singularity, a technological threshold after which computational systems are considered to have surpassed human abilities to think and reason, ushering a new civilizational epoch dominated by infinitely powerful non-biologic intelligence.
In recent years, technologists and computer scientists have made serious advances in Machine Learning (ML), the engineering field that underlies AI. Likewise, developments in large language models (LLMs), a class of deep learning architectures called transformer networks, and natural language processing (NLP), a subfield of AI that enables computers and digital devices to recognize and generate text and speech by combining computational linguistics with statistical modeling and vast troves of training data. These have given rise to systems that can effectively communicate with human language.
These include the now ubiquitous Chat agents like ChatGPT from OpenAI and Anthropic’s Claude, among others, that seem convincingly capable of fluent conversation, and even something uncannily resembling understanding and independent thought.
But are these systems genuinely intelligent or simply sophisticated statistical engines mimicking human language? How is ‘intelligence’ defined? Will we develop systems and agents that can act and intervene in human affairs responsibly and fairly? How can nation states, governments, investors, commercial enterprises, private actors, judiciaries, legislatures, and other powerful stakeholders reach practical consensus on and be held accountable to violations concerning complex matters of international cooperation and governance, privacy, regulation, and legal, ethical, and other critical protections against and responses to abuses, misuses, and other harms? What are the consequences if we don’t or can’t? Will AI’s risks outweigh the potential benefits?
Server Demirtaş, saklanan (hiding) 2018
Many of these and other related questions and issues are typically bundled as parts of the so-called alignment problem – the challenges of ensuring that the objectives and purposes of computational systems are compatible with and supportive of human values, goals, and preferences.
Researchers, engineers, designers, and programmers are tasked with building models and encoding and training systems with stringently clear purposes, objective functions, and safety constraints to ensure they will do the right thing, not figure out which thing is right. These are gargantuanly complex problems and entail countless conceptual, technical, behavioral, and even moral and ethical elements.
Can or will any of that be solved merely by keeping humans in-the-loop, as many proponents of a full-throttle AI push argue?
With or without resolution to these or any of the other hard problems or refinements in technical capability sufficient to produce true reliability, we are deep in an AI summer. AI is a juggernaut commercial sector with trillions of dollars at play. Deployment at scale will bring immense wealth, power, and influence to entrepreneurs, tech leaders, investors, and scores of other stakeholders. In ways large and small, it is recasting global industries, markets, and economies, impacting geopolitics, society, and child development, and altering the fabric of our daily lives.
Engineering Intelligent Machines
From AI’s earliest days, the project has been to engineer intelligent machines that could perform “the most advanced human thought activities.” It seems clear that today’s LLMs, conversational agents (ChatBots), and other algorithmic and machine learning-based applications and systems derive from that originating idea and are intended to credibly emulate human thinking and decision making. Claude disputed this when I prompted it about that, something I will write about, including Claude’s support for its counterarguments, in a future piece. Just the same and acknowledging AI systems are designed to use approaches to thinking-like processes and task-specific actions that may be intentionally different from human thought, I stand by my contention. The primary general models for AI are human cognition, decision-making, language use, and visual representations of people and physical artifacts.
But what are the sources of those models? Algorithmic design, training, and ML use-cases primarily draw from research grounded in computer science and mathematics, neuroscience, computational psychiatry, statistical linguistics, and other hard science analytics, together with frameworks about mentation and behavior based in psychometrics, and cognitive, behavioral, and social psychology, as well as vast tracts of data scraped from the internet. As Alison Gopnik’s work suggests, computational systems are being trained through the ingestion of super massive quantums of data, a process which departs in virtually every meaningful respect from how children acquire information, consolidate generalizable understandings about themselves and the world, and develop capacities for adaptational change over time and across a variety of situations.
What Turing and his many disciples pursuing the development of machines that can think have overlooked over the past seven decades is understanding how we think. Or more accurately put, understanding what thinking – which includes imputing and understanding meaning – is made of, not just delineating bio-mechanical processes servicing an output. One limitation of the foundational knowledge architecture underlying AI systems is over-reliance on cognition, rational decision-making, mechanistic and behavioristic conceptualizations and uses of language, and approximations of pseudo-mentation modelled on neural structures and cortical processes.
Human thought is, among many other things, constituted of the moment-by-momentness of living a life — the totality of subjective embodied experiences, whether recallable and remembered or not, involving the history of memory and emotion that comprise and immeasurably influence and aggregate into who we are and how each of us thinks and behaves.
Minimized or even left aside, in part because they often defy direct representation in language and, thus, statistical tangibility, are nuanced, inscrutable aspects of our internal worlds – the complex non-cognitive and affective dimensions of personality, and how we learn, think, and act. How each of us understands and operates in the world is an astronomically complex amalgam of hopes, fears, setbacks, successes, joys, heartbreaks, uncertainties, trauma, and relationships. All of which is riven with emotions and attached to clusters of personal history. None of that can be mathematically replicated in a computational simulacrum.
For all the anthropomorphizing, projection, self-referentialism, and human-like abilities these technologies are intended to resemble, the essential humanness on which they are based is close to non-existent.
AI is an umbrella term covering an array of computational systems, applications, services, and methods. Each provides or is intended to accomplish different things in various contexts. It can and can’t do certain things. But at its core, it’s a human enterprise devised, driven, and shaped not only by experimentation and innovation, but by our interests, hopes, fears, foibles, and fantasies.
With all of this in mind, those of us on The American Psychoanalytic Association’s Council on Artificial Intelligence, the CAI, think that psychoanalytic perspectives and expertise are relevant and needed in designing, developing, and deploying AI.
Enter The CAI Report. Our mission, and my role as Editor, is to offer psychoanalytic perspectives on a broad range of issues involving AI’s uses and applications, and risks and benefits to individuals and in society.
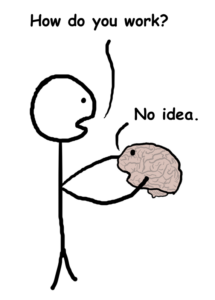
How Do You Work? by Tim Urban
Outcomes by Design or Unintended Consequences?
AI is a piece of human invention. But it’s being developed and introduced not just as a utilitarian tool. Many commercial AI systems don’t simply leverage computational potency in a conventional sense such as to analyze data, run interactive technologies, accelerate research and design problem-solving, or do other work for us with greater velocity, accuracy, and efficiency. Rather, their central functions are an inversion of those typical uses. There are now a plethora of systems designed to provide information about us (not for us), deliver data-driven guidance, and to monitor, assess, surveil, predict, manage, and influence–even manipulate–aspects of thought, emotion, and behavior both individually and at a societal level. Many of these applications and services are developed for the purpose of delivering what purports to be insight, feedback, reporting, and forecasting about what and how we think, feel, or behave. These include, for instance, affective computing, sentiment analysis, behavior change analytics, synthetic decision-making, and intelligent nudging, among others.
Added to these is a wave of mass-market applications purporting the capability of provisionally diagnosing and responding to depression, anxiety, relationship travails, and various other mental health issues. For instance, leapfrogging on the fluency and seeming sophistication of prompt responses contemporary systems deliver, there are now rafts of Chatbot “therapists.” These are, to be clear, not licensed mental health practitioners or even human beings, but machines. They were created, charitably, to help close the treatment gap – the shortage in public mental health systems where patient demand far outstrips the supply of available qualified clinicians. However, once private equity- and venture capital-backed tech companies recognized value opportunities in that gap, subscription-based text and video chat services were launched that enabled people to interact with a licensed therapist outside the conventional mental health care system.
Many of these AI therapeutic tools may have been developed to bring some form of mental health care to the masses. But as a practical matter, they are fraught with problems and risks. Some involve business models that drive growth and profit through lack of transparency to patients about how their ‘treatment’ will be conducted, and by making misleading claims about ‘gold-standards’ of ‘evidence-based’ therapy. I recommend The Psychotherapy Action Network’s Position Paper on Mental Health Apps/Technology (full disclosure, I’m a member of PSiaN’s Advisory Board) which outlines these emerging mental health tools and technologies including a list of red flags to identify problematic versions.
Other issues are encoded in the technology itself. The training data and algorithms embed fallacies and misconceptions around the origins and nature of certain psychological conditions and disorders; fundamentally misapply differential diagnostic best practices in non-organic scenarios; and are unequipped to discern and interpret presenting symptomatology, the root causes and substructures of which may be obliquely disguised by symbolic conversion, replacement, comorbidities, or involve nesting matrices of complex psychopathologies.
Social media and other digital technologies that are deeply embedded in our daily social communication and information networks are likewise susceptible to both unwitting and deliberate abuses and misuses of machine learning-based systems. In addition, user data – our most private and intimate details, not ‘just’ banking or other data signatures and credentials – are regularly shared with companies, institutions, data brokers, and other third-parties. Often, there’s no clear responsible party or agency for safeguarding the PII (Personally Identifiable Information) the systems harvest nor have robust legislative, legal, or regulatory mechanisms or AI data governance guidelines yet been broadly adopted to mitigate material and human risks or to provide equitable avenues for redress for harms caused.
The challenges and risks AI pose aren’t confined to large-scale global systems and data networks. Recent advances in GenAI’s capabilities have also given rise to new vectors of issues involving what Sherry Turkle discusses as the perils of pretend empathy and artificial intimacy. Not merely over-the-horizon conceptual challenges, these are currently iterated in real-world commercial ventures. There is already a robust profit-driven market sector producing sex-bots – sophisticated life-like artificial sex partners – and death-bots (also referred to as griefbots) – avatars that recreate the appearance, speech, and primary personality features of a deceased person.
And there is a growing body of social science research demonstrating that many AI-based systems and services are adversely impacting children’s psycho-social development and hamstringing kids’ abilities to learn and think critically, even as companies market and sell AI tools and services into the education and mental health marketplace. Similarly, many digital technologies that appeared (or were hoped) to be solutions to the adolescent and young adult mental health crisis following the Covid pandemic, are now being understood as causal to, not reparative of, increases in isolation, loneliness, anxiety, depression, self-harm, and suicidality.
Alarms have been sounding on the risks of prematurely integrating unsupervised automated technologies into the social fabric before thoroughly understanding and safeguarding against unintended consequences. A recently published Global Catastrophic Risk Assessment Report from the U.S. Homeland Security Operational Analysis Center advises that “AI systems have the potential to destabilize social, governance, economic, and critical infrastructure systems, as well as potentially result in human disempowerment” and can amplify “existing catastrophic risks, including risks from nuclear war, pandemics, and climate change.”
How did we get here? Arguably, the most outsized influences behind these approaches to leveraging emerging technologies come from the principal innovators, tech and business leaders, and investors whose personal beliefs and psychological proclivities are guiding everything, from concept, design, research, and development, to functional execution and commercialization. Many of them subscribe to a bundle of ideologies known as TESCREAL, an acronym coined by Émile Torres which denotes “transhumanism, Extropianism, singularitarianism, (modern) cosmism, Rationalism, Effective Altruism, and longtermism.” At the heart of TESCREALism, as Torres explains, is a “techno-utopian” vision of the future.
Supporting the TESCREAList vision are armies of technologists, computer scientists, researchers, engineers, and other technical professionals at the frontlines of design, development, training, and testing. Whether ideologically aligned or not, their personal beliefs, biases, values, and psychologies are unavoidably implicated in what they contribute to and produce.
But AI is also profoundly shaped by all of us, the end-users. Much of AI’s high-velocity ascent, separate from what it can or can’t actually currently do, is driven by our collective acceptance of its anthropomorphized presentation. All of this has been enabled by the combined forces of the technology industry’s and the media’s relentless delivery of marketing propaganda masquerading as validated science. And by constantly presenting words and images that bond together the idea of AI as equivalent to the human brain.
We see this, for example, in “Hallucinations,” a term referring to plausible-sounding AI-generated mistakes, falsehoods, or absurdities. But hallucinations are the false perception of objects or events involving our senses—a perception without a stimulus—the typical causes of which are neuro-chemical or neuro-structural abnormalities or psychological trauma. Human brains and minds hallucinate. Computer programs do not.
Similarly, an entire generation is coming of age brain-washed by an emoji-based propaganda campaign using brain-shaped clouds, brains overlaid with circuit-boards or spinning electrons, heads containing computers instead of brains, robots that look like a person thinking, and other pictograms and iconography signifying that artificial intelligence, machine cognition, and the human brain are interchangeably synonymous.
The net effect of this is that we have willingly allowed a powerful but still largely unproven technology to be integrated into nearly every strata of society, commerce, and human affairs.
The history of technology is a compendium of both wondrous constructive advances alongside devastating unintended consequences. But AI stands apart in the volume and intensity of delirious hype and feverish overestimations of prowess. Much of what makes its way into social media feeds and mainstream news sources tends to inflame catastrophizing prognostications, alternately generate hysteria, reverence, or consternation around machine consciousness, algorithmic motivational autonomy, agentic independence, and quasi-religious paroxysms portending human subjugation, extinction, or both.
There is ample commentary on the ways AI is already adversely impacting contemporary life: algorithmic bias, wide-scale disinformation and social manipulation, the pillage of privacy, the weaponization and profiteering of personal data, intrusive apartheid-esque surveillance, impingements on cognitive liberty, deep fakes and the erosion of verifiable objectivity created by a deluge of synthetic slop and plausibly credible but actually incorrect data; as well as fraud, corruption, and cybercrime on digital steroids, and the generation of myriad moral, ethical, legal, and socio-political dilemmas of gargantuan proportions.
Many critics, while cautiously enthusiastic about AI’s theoretical potential and acknowledging some of its impressive accomplishments, are unswayed by the hype. Far from lauding these systems as prodigious intellectual leviathans, they characterize them as Stochastic parrots – automatons incapable of exercising common sense, comprehending meaning, or developing genuine understanding in any human sense of the concept.
Others importantly underscore what AI is and is not, and – to borrow from the title of Selmer Bringsjord’s still prescient 1992 book essaying why AI is incapable of producing robotic persons or computational systems that could have free will and make moral decisions – what it can and can’t be.
Beyond the inflammatory propaganda, zealotry, and howls of peril, there are pockets of genuine promise. Some current systems demonstrate exceptional and useful capabilities, and in many commercial sectors the value proposition is clear. The positive impact of the compute and analytic power directed toward problem solving and innovation, among other application areas, is already established in many branches of science, technology, and engineering. These suggest that the alignment problem and other risks and social disruptions could be lessened if more effort were expended in leveraging AI’s true potential to help us and less in debating machine consciousness and trying to replicate human-like thought.
With that in mind, a final thought before introducing The CAI Report’s guiding principles and the initial group of pieces.
Michael I. Jordan, a leading researcher in AI and machine learning and a professor in the department of electrical engineering and computer science at the University of California, Berkeley, notes that the imitation of human thinking should not be the sole or even the best goal of machine learning. Instead, as the first engineering field that is humancentric, focused on the interface between people and technology, he thinks it should serve to augment human intelligence and provide new services.
“While the science-fiction discussions about AI and super intelligence are fun, they are a distraction,” Jordan says. “There’s not been enough focus on the real problem, which is building planetary-scale machine learning–based systems that actually work, deliver value to humans, and do not amplify inequities.”
We at The CAI Report agree.
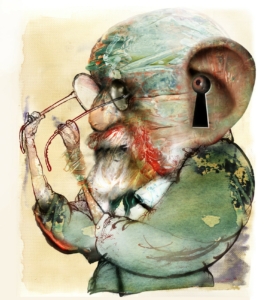
Distaste for Sigmund Freud by Horacio Cardo
The CAI Report–At the Intersection of Psychoanalysis and Artificial Intelligence
One of our aims is for The Report to be a respected go-to resource where folks in the technology space and the general public—not just psychoanalysts, psychiatrists, and other mental health professionals—can learn about how psychoanalysts think about and approach issues relating to emerging technologies like AI. And not incidentally bust a few myths and correct some misunderstanding about psychoanalysis along the way.
We also hope The Report will attract the attention and interest of tech leaders, investors, policymakers, computer scientists and other professionals involved in designing and developing AI systems with a view, to paraphrase Lin-Manuel Miranda in “Hamilton”, to being invited into the rooms where things happen. Psychoanalysts have uniquely important things to say about the human and social dimensions of AI.
Psychoanalysis is a specialist discipline of thinkers and practitioners with core expertise and competencies in both understanding the complexity of the human condition and pragmatically treating mental and emotional problems and issues deriving from our developmental, relational, and emotional histories. Through extensive education, training, and more than a century of aggregated theory-building and validated clinical data, psychoanalysts are peerless in making sense of seemingly inscrutable features of individual and collective experience and decision-making. Psychoanalysts are also experts in identifying how our affects – emotions – combine with personal history and memory to function as integral to cognition – thinking – which in turn triggers our behavior.
Everyone will benefit when we can bring that to bear in influencing how gap-leaping technologies like AI are developed and applied in society. Examining the role of emotions in cognitive processes, for instance, is an especially useful though largely undervalued and unexplored topic in AI research in which psychoanalysis has unmatched expertise.
Journalistic balance and fairness are also important considerations. Will, or should, content in The Report take a position on AI or remain generally neutral? My view as Editor is that we ought to bring an analytic attitude – open-minded, questioning, keenly attentive – to opening AI’s black box, using domain-specific knowledge and expertise to illuminate what’s working and why, while furthering the principles of the likes of Edward R. Murrow in surfacing what’s not right and holding people in positions of power and influence accountable. These can and should be offered with candor, equanimity, and scientific discipline but also without pulling any punches.
Our contributors will be psychoanalysts with knowledge about and fluency in technology. Some will maintain a generally neutral stance while some may be unapologetically optimistic about AI’s potential. Others, like me, will offer skeptical views and may skew more toward criticism, concern, or caution than enthusiasm. But we will all deliver incisive commentary on issues around the complex interplay between technology and the mind, how we relate to and are affected by the tech, and how our imaginings about AI influence not just our uses of it but how we see and experience ourselves. The Report’s content will, we hope, be thoughtful, thought-provoking, and well-written, engaging, and adhere to scientific and psychoanalytic standards of ethics and evidentiary supportability.
This inaugural issue begins with a Note from Todd Essig, PhD and Amy Levy, PsyD, the Founders and Co-Chairs of the Council on Artificial Intelligence.
In a segment called “Useful Reads” which will offer a brief overview or review of a recent publication, article or book at the intersection of psychoanalysis and AI, Todd Essig provides his thoughts, with computational ‘collaboration’ from Anthropic’s Claude, on “Narcissistic Depressive Technoscience” by Thomas Fuchs which appeared in the Spring 2024 volume of The New Atlantis.
Amy Levy shares a short excerpt from a chapter of her forthcoming book, “The New Other: Alien Intelligence and The Innovation Drive.”
Next, Valerie Frankfeldt, PhD offers “Artificial Intelligence and Actual Psychoanalysis,” a summary and commentary of a CAI Workshop delivered on July 21, 2024 with presenters Amy Levy, PsyD, Danielle Knafo, PhD, and Todd Essig, PhD, along with AI Ada, an avatar discussant; and Fred Gioia, MD, moderator.
In what we anticipate will be a recurring series exploring cutting edge research and clinical applications of artificial intelligence in mental health treatment, Frontiers in AI & Mental Health: Research & Clinical Considerations, Christopher Campbell, MD Candidate (Medical University of South Carolina), presents the latest translational AI research to provide tools to better understand how AI is impacting mental health, inspire ideas for integrating these advances into clinical practice, and spark curiosity about the future innovations that lie ahead. His first entry (with assistance from ChatGPT4o/Scholar AI in summarizing the research studies) is “Leveraging AI for Suicide Risk Prediction.”
AI and mental health is too broad a lane for a single column. Be on the lookout for additional entries that dive into identifying, understanding, and determining how clinicians and society can respond to the expanding taxonomies of techno-pathology not yet covered in the DSM, such as how AI is changing our minds, and how we love and relate.
Looking ahead, we plan to launch a series of interviews. These will feature notable folks who are leading, influencing, and innovating the development of AI-related systems, applications, and services as well as other change-makers and expert voices in the space in conversation with a tech-savvy psychoanalyst. We’ll also include an ‘interview’ of an AI conversational agent. As we generate a pipeline of compelling interviewees, please send your suggestions to apsa.cai.report@gmail.com.
The interactional nature of AI is a key feature of the technology. It’s not enough to talk about them; we also need to engage with them. We will publish prompt-and-response ‘conversations’ with various large language model agents. These won’t just be raw transcripts but will be annotated with psychoanalytic commentary. We’ll cover questions and issues at the intersection of psychoanalysis and technology, such as the one I noted in which I prompted Claude about the primary aim of AI, the main differences between how humans and AI systems make decisions, and how expertise in human thought, such as from psychoanalysis, can assist with the alignment problem. I also prompted Claude around issues relating to symptom diagnosis and treatment recommendations for various mental health issues. That opened into a deeper ‘discussion’ on ways AI systems could benefit from direct input by mental health experts, including psychoanalysts, as well as probing the real-world implications in a number of applications and services of AI’s incapacities with empathy, emotion, symbolic inference, and limitations in how computational systems can meaningfully interface with human experiences and psychological complexity.
The CAI has a team examining the intersection of AI with child and adolescent development. They’ve begun studying how socio-emotional development is already being influenced by AI and we look forward to presenting reports on their findings and recommendations.
No discussion of children and adolescents can exclude education, an area in which AI is transforming (or deforming) how teachers teach, how students learn, and even prompting reconsiderations about the nature of education itself. You can expect to read pieces on these topics along with discussions on courses and curricula being developed at all stages, from elementary to graduate school, addressing and responding to the influences of technology in the classroom.
We’re also looking to roll out a series on problems and solutions in respect of a range of what we’re provisionally calling Impact Zones — children, adolescents, the elderly, marginalized social groups, mental health, ethics, law, cognitive liberty, business and work, the environment, and a host of other industrial, social, and economic areas where these systems are or will affect people and society.
In addition to Useful Reads, we will periodically include pieces commenting on films, theater, and other works from culture and the arts in which AI is the dominant subject. We’ll also spotlight recent developments in the AI space with psychoanalytically inflected commentary on them. And we’ll offer a curated menu of upcoming events and conferences as well as a select roster of noteworthy books, articles, podcasts, videos, research, and other content recommended by CAI members. Please be in touch with your recommendations for events and resources we might include or if you know about a conference at which a CAI member should be a speaker. Contact us at apsa.cai.report@gmail.com.
In a landscape glutted with content options, we hope you’ll find The CAI Report worth your valuable time. Thank you for joining us.